The underpinnings of human cognition remain mostly a mystery. Despite the advancement of tools and technology in neuroscience, our understanding of the brain and its functions is pretty superficial. Part of the problem lies in how we describe neuroscientific phenomena. The metaphors neuroscientists commonly use are taken from communications fields; they borrow terms such as “processing stage” and “information transfer”. However, these analogies only take us so far, and they aren’t close enough to real biology. Daniel Bear argues that we need to start describing neuroscience in the language of a field inspired by evolution: machine learning.
Brains are not designed exactly in the way that information theory demonstrates them to be. It’s not all wrong, as the brain does follow computational principles in its transformations from stimuli to behaviour. However, the principles we use right now don’t fit perfectly. We haven’t improved on many of the early models. Through a machine learning process, an algorithm is learned automatically using a given rule to which the machine can adjust its internal parameters. Then slowly, through trial and error and internal adjustments, the machine learns all on its own.
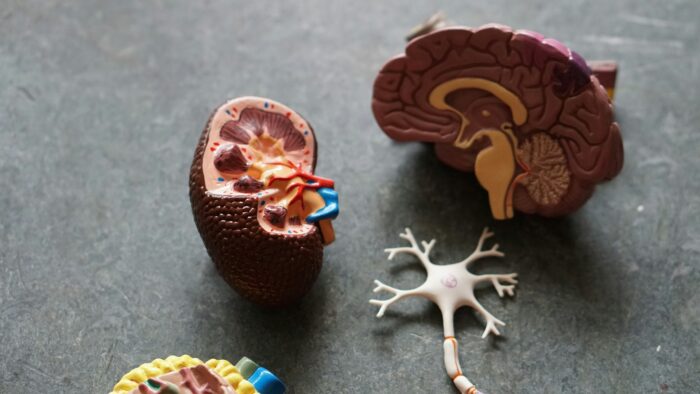
Photo by Robina Weermeijer on Unsplash.
Through a similar process to natural selection, our brains may have learned how to use stimuli and produce behaviour by taking small steps in the right direction until perfecting the process. The defined areas of the brain and specific neurons serving different functions are there because they work. The brain adapted to function the way it does. Therefore, it follows that the best way to understand the functions of the human brain is through the lens of the simultaneously computational and evolutionary field of machine learning. This new perspective does not mean that we will finally understand everything about the brain, but just like evolution, it will point us in the right direction.
Tags: brain, learning, machine learning, scienceCategorised in: Uncategorized
This post was written by McKenzie Cline